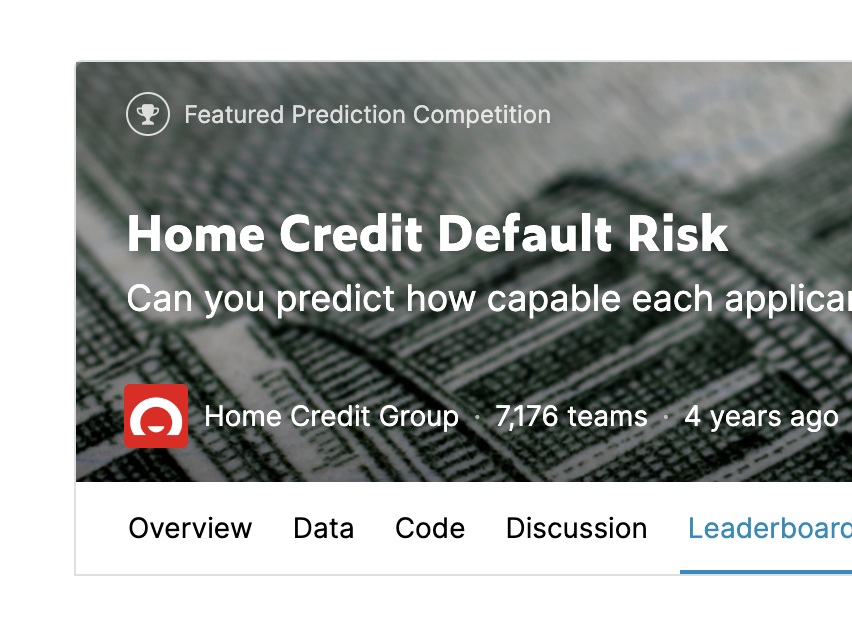
https://www.kaggle.com/competitions/home-credit-default-risk/data
Home Credit Default Risk | Kaggle
www.kaggle.com
데이터 모델 설명
- 메인 테이블 : application_{train|test}.csv
- 고객의 정보와 현재 대출에 대한 정보를 제공한다.
- 1이면 미납자, 0이면 성실한 사람
- SK_ID_CURR
- 과거 대출 이력 : previous_application.csv
- 고객의 현재 대출 이전의 과거 대출 정보를 제공한다.
- SK_ID_PREV, SK_ID_CURR
- 타사 대출 이력 : bureau.csv
- 고객의 현재 대출 이전의 타사 대출 정보를 제공한다.
- SK_ID_BUREAU, SK_ID_CURR
- 타사 대출 월별 잔액 : bureau_balance.csv
- Monthly balances of previous credits in Credit Bureau.
- This table has one row for each month of history of every previous credit reported to Credit Bureau – i.e the table has (#loans in sample * # of relative previous credits * # of months where we have some history observable for the previous credits) rows.
- 과거 대출의 월별 현금 대출 잔액 : POS_CASH_balance.csv
- Monthly balance snapshots of previous POS (point of sales) and cash loans that the applicant had with Home Credit.
- This table has one row for each month of history of every previous credit in Home Credit (consumer credit and cash loans) related to loans in our sample – i.e. the table has (#loans in sample * # of relative previous credits * # of months in which we have some history observable for the previous credits) rows.
- 과거 대출의 월별 카드 대출 잔액 : credit_card_balance.csv
- Monthly balance snapshots of previous credit cards that the applicant has with Home Credit.
- This table has one row for each month of history of every previous credit in Home Credit (consumer credit and cash loans) related to loans in our sample – i.e. the table has (#loans in sample * # of relative previous credit cards * # of months where we have some history observable for the previous credit card) rows.
- 과거 대출의 월별 납부 이력 : installments_payments.csv
- Repayment history for the previously disbursed credits in Home Credit related to the loans in our sample.
- There is a) one row for every payment that was made plus b) one row each for missed payment.
- One row is equivalent to one payment of one installment OR one installment corresponding to one payment of one previous Home Credit credit related to loans in our sample.
- HomeCredit_columns_description.csv
주요 분석 도메인 : 고객 자산, 고객 소득, 고객 거주지, 고객의 행동, 타기관 대출 이력, 과거 대출 납부 이력, 과거 대출 이력, 고객 신상(성별, 직장 유무), 대출 금액, 신용 점수 등
application_train(test) 시각화 및 Preprocessing
Data load
import numpy as np
import pandas as pd
import gc
import time
import matplotlib.pyplot as plt
import seaborn as sns
#import warning
%matplotlib inline
#warning.ignorewarning(...)
pd.set_option('display.max_rows', 100)
pd.set_option('display.max_columns', 200)
app_train = pd.read_csv('/home/soon5770/Kaggle/home-credit-default-risk/application_train.csv')
app_test = pd.read_csv('/home/soon5770/Kaggle/home-credit-default-risk/application_test.csv')
app_train.head()
TARGET 값에 따른 AMT_INCOME_TOTAL값 분포도 비교
- distplot과 violinplot 시각화
- plt.subplots() 기반으로 seaborn의 distplot과 violinplot으로 분포도 비교 시각화
cond1 = (app_train['TARGET']==1)
cond0 = (app_train['TARGET']==0)
cond_amt = (app_train['AMT_INCOME_TOTAL']<500000)
sns.distplot(app_train[cond0 & cond_amt]['AMT_INCOME_TOTAL'], label='0', color='blue')
sns.distplot(app_train[cond1 & cond_amt]['AMT_INCOME_TOTAL'], label='1', color='red')
sns.violinplot(x='TARGET', y='AMT_INCOME_TOTAL', data=app_train[cond_amt])
한 번에 그리기
fig는 전체 그림을 말하고, axs는 각각의 subplot의 축을 말한다.
위의 코드에 ax 지정만 해주면 된다.
cond1 = (app_train['TARGET']==1)
cond0 = (app_train['TARGET']==0)
cond_amt = (app_train['AMT_INCOME_TOTAL']<500000)
fig, axs = plt.subplots(figsize=(12,4), nrows=1, ncols=2, squeeze=False) # 1행 2열의 subplot 만들기
sns.violinplot(x='TARGET', y='AMT_INCOME_TOTAL', data=app_train[cond_amt], ax=axs[0][0])
sns.distplot(app_train[cond0 & cond_amt]['AMT_INCOME_TOTAL'], label='0', color='blue', ax=axs[0][1])
sns.distplot(app_train[cond1 & cond_amt]['AMT_INCOME_TOTAL'], label='1', color='red', ax=axs[0][1])
위 코드를 함수화 해보자
def show_column_hist_by_target(df, column, is_amt=False):
cond1 = (df['TARGET'] == 1)
cond0 = (df['TARGET'] == 0)
fig, axs = plt.subplots(figsize=(12,4), nrows=1, ncols=2, squeeze=False) # 1행 2열의 subplot 만들기
cond_amt = True
if is_amt:
cond_amt = df[column] < 500000
sns.violinplot(x='TARGET', y=column, data=app_train[cond_amt], ax=axs[0][0])
sns.distplot(app_train[cond0 & cond_amt][column], label='0', color='blue', ax=axs[0][1])
sns.distplot(app_train[cond1 & cond_amt][column], label='1', color='red', ax=axs[0][1])
show_column_hist_by_target(app_train, 'AMT_INCOME_TOTAL', is_amt=True)
app_train과 app_test를 합쳐서 한번에 데이터 preprocessing 수행
train data에 데이터를 추가할건데 또 같은 로직으로 test data를 만들기 귀찮기 때문에 train data와 test data를 합친다.
그리고 다시 분리한다.
target이 있는 데이터가 train data이고, target이 없는 데이터가 test data 이다.
app_train.shape, app_test.shape
((307511, 122), (48744, 121))
apps = pd.concat([app_train, app_test])
apps.shape
(356255, 122)
Object feature 들을 Label Encoding
Light GBM 같은 추리계열에서는 Label Encoding이 더 좋다.
pandas의 factorize() 이용 : 한번에 한 컬럼만 적용이 가능하므로 루프를 돌려야 한다.
apps.dtypes[apps.dtypes == 'object']
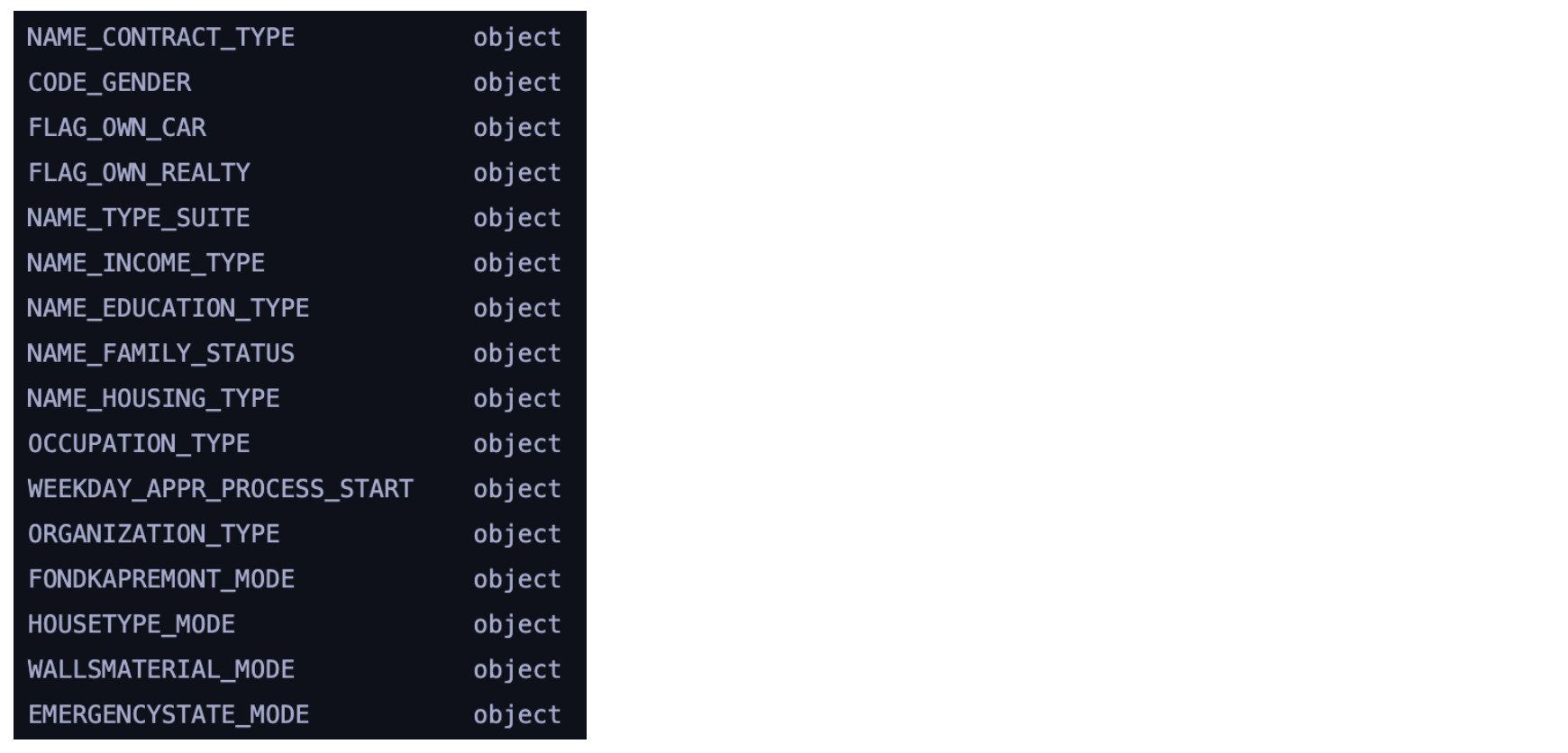
object_columns = apps.dtypes[apps.dtypes == 'object'].index.tolist()
for column in object_columns:
apps[column] = pd.factorize(apps[column])[0] # 앞에것을 가져오므로 [0]
Null 값 일괄 변환
null 값 확인하기
apps.isnull().sum().head(100)
-999로 모든 컬럼들의 Null값 변환
apps = apps.fillna(-999)
다시 null 값을 확인하면
null 값이 없는 걸 확인할 수 있다.
train, test 데이터 다시 분리
app_train = apps[apps['TARGET'] != -999]
app_test = apps[apps['TARGET'] == -999]
app_train.shape, app_test.shape
((307511, 122), (48744, 122))
잘 분리 되었지만 여기서 test 데이터는 target 값이 떨어져 나가야 한다.
app_test = app_test.drop('TARGET', axis=1, inplace=False)
app_test.shape
(48744, 121)
.
'💡 AI > Dacon | Kaggle' 카테고리의 다른 글
kaggle notebook의 첫 코드는 뭘까? (0) | 2022.03.27 |
---|---|
작물 병해 분류 AI 경진대회 (1) | 2021.10.16 |