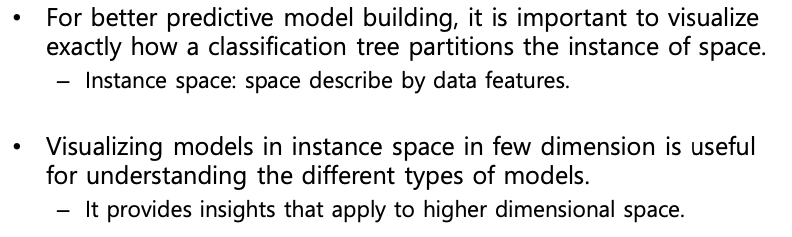
Visualizing Segmentations
데이터를 Age라는 변수로 나눈다.
entropy와 Information gain을 기준으로 나눈다.
x축 : Age
y축 : Balance
어떤식으로 Decision boundary가 결정되었는지 알 수 있다.
여기서 어떻게 바운더리를 설정해야 정확성을 유지할 수 있을까?
Geometric interpretation of a model
아무리 잘해도 완벽하지 않다.
의사결정트리 말고 어떤 다른 방법으로 자를 수 있을까?
줄을 하나 그음으로써 Classification이 달라질 수 있다.
Trees as Sets of Rules
의사결정 트리는 rule set과 같다.
Trees as Sets of Rules
What are we predicting?
빨간 데이터는 life insurance에 관심이 있느냐 없느냐에 No라는 답이 나왔다.
MegaTelCo: Predicting Customer Churn
확률을 앎으로써 고객이 떠나지 않게 할 수 있으므로
확률에 따라서 예산을 확보하고 다양한 비지니스적인 일을 할 수 있다.
From Classification Trees to Probability Estimation Trees
overfitting을 낮추려면..?
Laplace Correction
라플라스 보정으로 확률 값에 조금더 신뢰성을 줄 수 있다.
보정이 있었을 때(점선) converge 되는 것을 볼 수 있다.
The many faces of classification:
Classification / Probability Estimation / Ranking
Ranking : 선호도 부여
Probability estimation : 확률 부여
Ranking은 서로 상호적인 것이다.
확률을 부여하는 것은 굉장히 어려운 일이다.
그래서 가정이라는 것을 한다. assumption
Let’s focus back in on actually mining the data..
MegaTelCo:
Predicting Churn with Tree Induction
Information gain 후 가장 높은 순으로 나와있다.
이 순서대로 우리가 tree를 만들어 나가면 된다.
테이블 순서대로 되지 않았다.
테이블은 서로의 관계성을 고려하지 않고 얻은 information gain이다.
트리를 구성할 때는 서로의 관계성을 고려하여 얻은 것이다.
overfitting을 막으면서.
'💡 AI > ML' 카테고리의 다른 글
ML W5-1 Fitting a Model to Data [OO] (0) | 2021.03.29 |
---|---|
ML W4-1-2 Fitting a Model to Data (0) | 2021.03.22 |
ML W3-2-2 - Supervised Segmentation (0) | 2021.03.18 |
ML W3-2-1 Supervised Segmentation (0) | 2021.03.18 |
ML W3-1-2 - Predictive Modeling (0) | 2021.03.15 |